data has become a critical component of our lives. We generate, consume, and process data on an unprecedented scale. But, what happens when the data becomes too large, complex, or varied? This is where Big Data Analytics comes into play.
Understanding Big Data
Before we delve into the world of Big Data Analytics, it’s crucial to grasp the concept of Big Data itself. Big Data refers to massive datasets that are too large, diverse, or complex for traditional data processing systems to handle.
Characteristics of Big Data
Big Data is often characterized by the “5 Vs”: Volume, Velocity, Variety, Veracity, and Value.
Volume
Volume refers to the sheer size of the data. With the rise of the internet, social media, and IoT devices, data volumes are growing exponentially.
Velocity
Velocity refers to the speed at which data is generated, processed, and analyzed. As technology advances, the rate at which data is produced and processed accelerates as well.
Variety
Variety refers to the different types of data, including structured, semi-structured, and unstructured data. It encompasses everything from text and images to audio and video files.
Veracity
Veracity refers to the accuracy and reliability of the data. In the world of Big Data, ensuring that the data is accurate and trustworthy is crucial for making informed decisions.
Value
Value represents the potential benefits that can be derived from the data. Big Data has immense value, but it’s crucial to extract meaningful insights through analytics to unlock its full potential.
Big Data Analytics
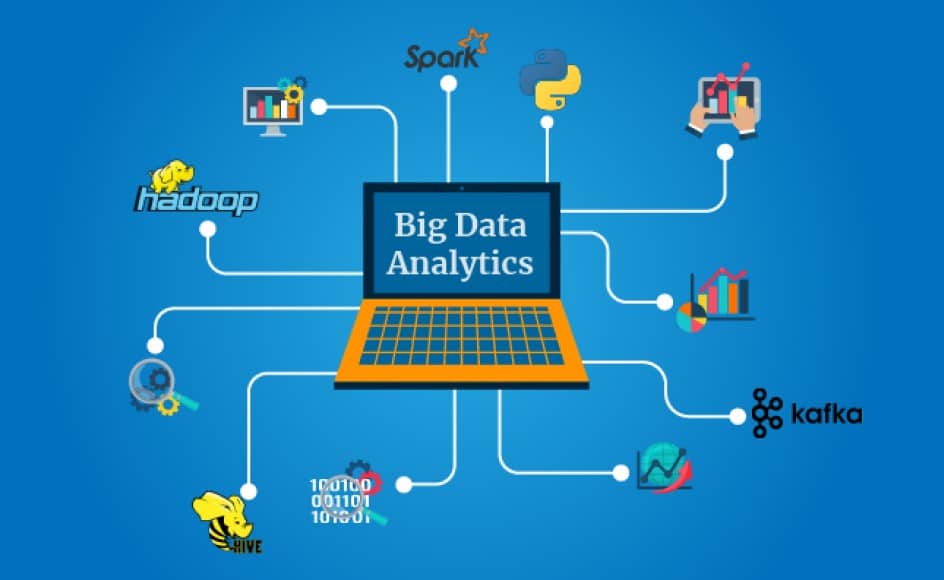
Big Data Analytics is the process of examining, cleaning, transforming, and modeling large datasets to uncover hidden patterns, correlations, trends, and other useful insights. These insights can help businesses and organizations make better decisions, improve efficiency, and gain a competitive edge.
Types of Big Data Analytics
There are four primary types of Big Data Analytics:
Descriptive Analytics
Descriptive Analytics focuses on analyzing past data to understand what has happened. It provides insights into historical patterns and trends.
Diagnostic Analytics
Diagnostic Analytics delves deeper into the data to determine the root causes of specific events or issues. It helps identify patterns and relationships that might explain past occurrences.
Predictive Analytics
Predictive Analytics uses historical data to forecast future events or trends. It enables businesses to make data-driven decisions and better prepare for potential challenges.
Prescriptive Analytics
Prescriptive Analytics goes beyond predicting future outcomes by suggesting actionable steps to optimize those outcomes. It helps businesses and organizations make informed decisions and take proactive measures.
Tools and Technologies
Several tools and technologies have emerged to facilitate Big Data Analytics, including:
Hadoop
Hadoop is an open-source framework designed for distributed storage and processing of large datasets. It allows businesses to store, manage, and analyze massive amounts of data using a cluster of computers.
NoSQL Databases
NoSQL databases, such as MongoDB and Cassandra, provide an alternative to traditional relational databases for managing unstructured or semi-structured data. They offer scalability, flexibility, and high performance, making them ideal for Big Data applications.
Machine Learning Frameworks
Machine Learning frameworks, such as TensorFlow and Apache Spark, enable data scientists to develop predictive models and algorithms that can learn from data and improve over time. These frameworks are essential for harnessing the power of Big Data to make accurate predictions and optimize decision-making.
Applications of Big Data Analytics
Applications across various industries and sectors, including:
- Healthcare: Identifying patterns and trends in patient data to improve patient outcomes and reduce healthcare costs.
- Finance: Detecting fraudulent activities, managing risk, and optimizing investment strategies.
- Retail: Personalizing customer experiences, optimizing inventory management, and improving supply chain efficiency.
- Manufacturing: Enhancing product quality, reducing downtime, and streamlining production processes.
- Transportation: Optimizing route planning, predicting maintenance needs, and improving traffic management.
Challenges in Big Data Analytics
Despite its immense potential, faces several challenges, such as:
- Data privacy and security concerns
- Data integration and quality issues
- Lack of skilled professionals
- High costs associated with infrastructure and processing
- Complexities in managing and analyzing massive datasets
Conclusion
Big Data Analytics has revolutionized the way businesses and organizations operate, providing valuable insights and enabling data-driven decision-making. By understanding and embracing the potential, businesses can unlock the full value of their data and gain a competitive edge in the rapidly evolving digital landscape.
FAQs
- What is Big Data Analytics? s the process of examining, cleaning, transforming, and modeling large datasets to uncover hidden patterns, correlations, trends, and other useful insights.
- What are the 5 Vs of Big Data? The 5 Vs of Big Data are Volume, Velocity, Variety, Veracity, and Value.
- What are some tools and technologies used in Big Data Analytics? Some tools and technologies used in Big Data Analytics include Hadoop, NoSQL databases, and Machine Learning frameworks.
- What are some applications of Big Data Analytics? Applications across various industries, including healthcare, finance, retail, manufacturing, and transportation.